Operational Technology (OT) environments, traditionally prioritized availability and physical safety, are significantly transforming. With the growing integration of IT and OT systems, adopting Artificial Intelligence (AI) and Machine Learning (ML) has become a game-changer in enhancing security. These technologies enable proactive threat detection, predictive maintenance, and anomaly detection, helping organizations mitigate potential risks in real time.
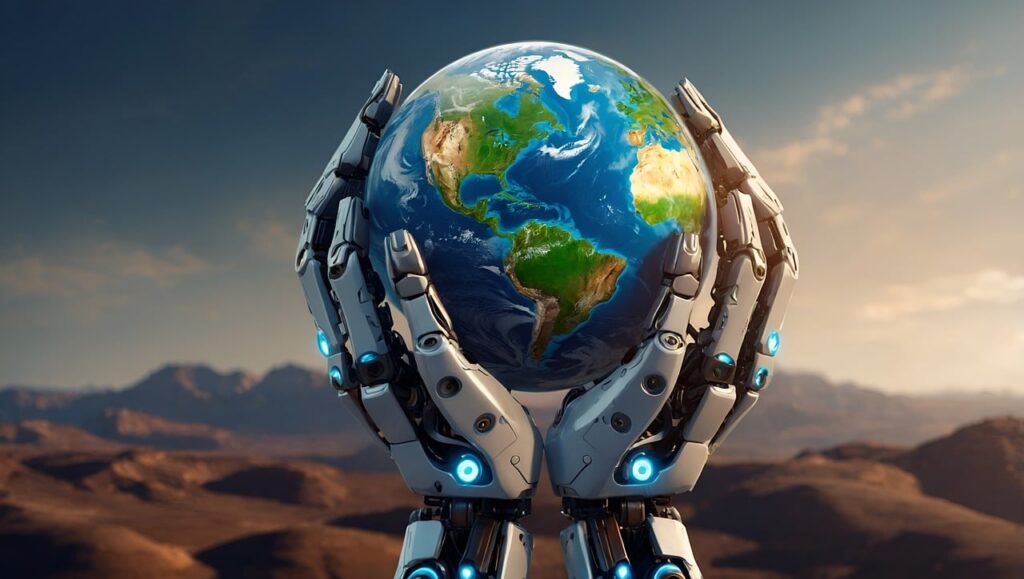
The Role of AI and ML in OT Cybersecurity
- Enhanced Predictive Analysis:
AI and ML can analyze large volumes of data generated by OT devices, identifying patterns and anomalies that might signal potential security threats. By predicting these issues, organizations can implement preventive measures before damage occurs. - Anomaly Detection:
Traditional security systems often rely on static rules, making them less effective against evolving threats. ML models, trained on historical and real-time data, can dynamically detect unusual activities, such as unauthorized access or system misconfigurations. - Improved Incident Response:
AI-powered tools can quickly identify and prioritize critical incidents, enabling faster response times. This minimizes downtime and reduces the potential impact of a cyberattack. - Automation of Routine Tasks:
AI and ML automate repetitive security tasks, such as log analysis and vulnerability scans. This not only improves efficiency but also allows human resources to focus on strategic decision-making.
Benefits of AI and ML Adoption in OT Environments
- Real-Time Monitoring: Continuous analysis of OT systems ensures early detection of anomalies.
- Proactive Risk Mitigation: Predictive models reduce the likelihood of system failures and security breaches.
- Reduced False Positives: AI and ML algorithms learn from past data, improving the accuracy of threat detection.
- Scalability: AI-driven solutions can scale alongside OT infrastructure, accommodating the growing complexity of industrial systems.
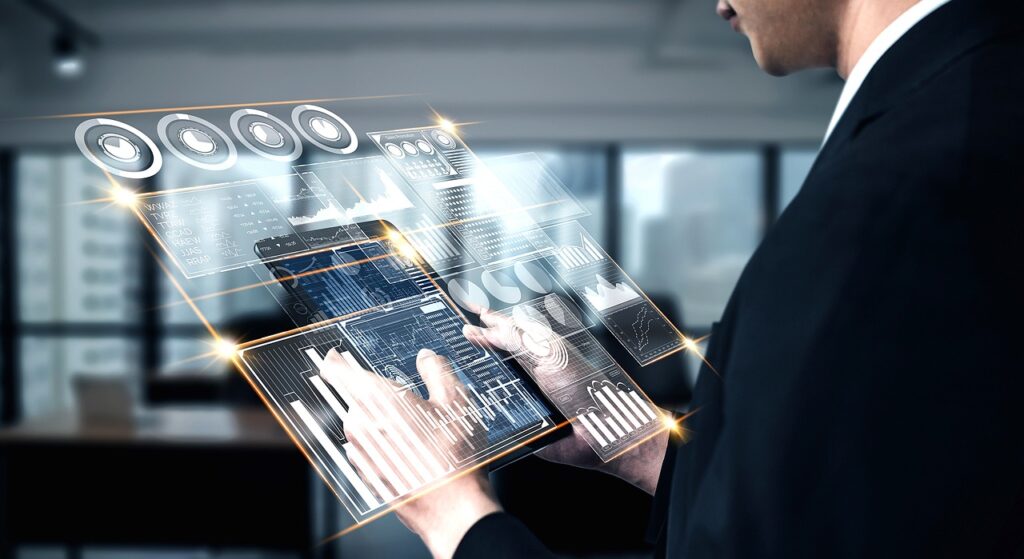
Challenges in Implementation
- Data Quality: AI and ML models require large datasets to perform effectively. Poor data quality can lead to inaccurate predictions.
- Integration Complexities: Many OT systems use legacy equipment, making integration with modern AI tools challenging.
- Skill Gaps: The implementation of AI and ML requires specialized expertise, which is often lacking in traditional OT teams.
- Cybersecurity Risks: AI systems themselves can become targets for cyberattacks if not adequately protected.
Emerging Use Cases
- Energy Sector: AI-driven systems monitor and optimize grid performance, ensuring uninterrupted power supply.
- Manufacturing: Predictive maintenance powered by ML prevents equipment failures, saving costs and enhancing productivity.
- Healthcare: AI monitors critical OT systems in medical facilities to ensure patient safety and data security.
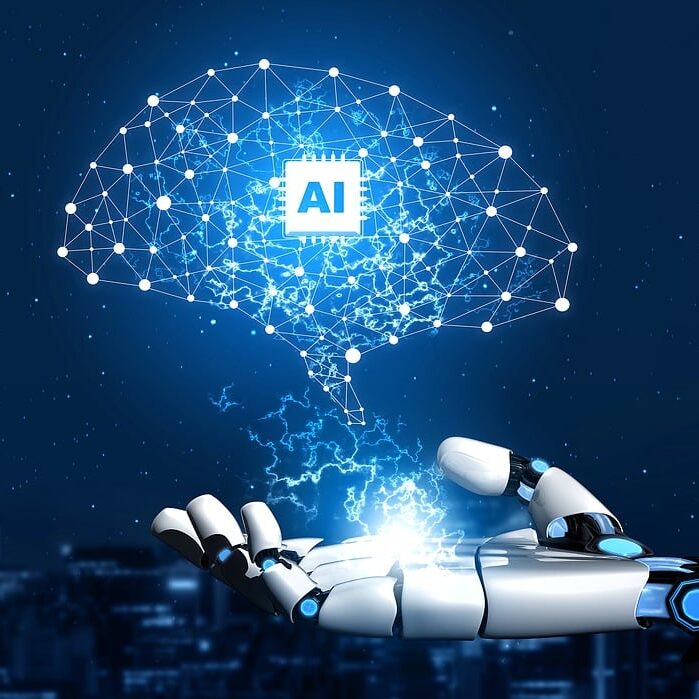
References and Useful Resources
- National Institute of Standards and Technology (NIST)
- ISA/IEC 62443 Standards for OT Security
- MIT Technology Review: AI in Industry
- Gartner Research on AI and OT
AI and ML are no longer optional in OT environments; they are essential for ensuring security and operational resilience. By leveraging these technologies, organizations can stay ahead of threats, enhance efficiency, and future-proof their industrial operations.
Stay Connected for more updates!